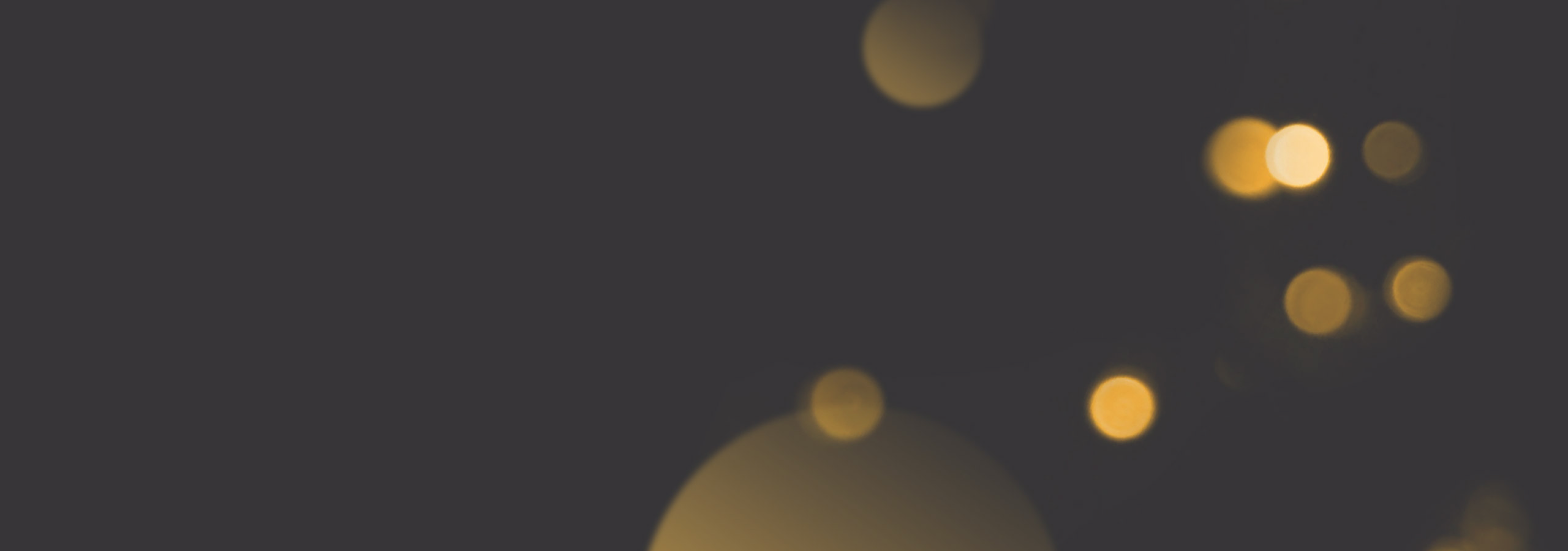
Many organisations assume that having a data lake means that there is no longer any need for a data warehouse. Nothing could be further from the truth; the one does not exist without the other. What is more, data lakes and data warehouses reinforce one another. But what is the difference and how do organisations get more out of their data by combining both applications?
The difference between a data lake and a data warehouse can be explained fairly simply. Briefly, it comes down to the fact that a data warehouse fulfils the need for structured and delineated data storage, above all providing clarity about output and results. In a data lake, both structured and unstructured data can be stored and various types of analytics can be carried out on this basis, such as in the field of visualisation, big data processing, real-time analytics and machine learning. With a data lake, an on the fly structure can be put in place and information requirements can be met more flexibly. To make optimal use of data, it is important that a data warehouse builds on a data lake and vice versa. The following four steps provide pointers here.
Step 1: Analyse the existing situation
As soon as an organisation has opted to embark upon a digital transformation, the first step is to look at the existing operating processes. What information is already available within the organisation and can therefore be included in a data lake? And how could this information be further enriched? During this phase, try to formulate the goals as clearly as possible so that it is obvious which datasets will be necessary for this. This first phase is one of experimentation, looking to see where improvements can be made in the process and how data can offer added value here.
Step 2: Develop a proof of concept
In this second phase, data are imported and injected. Or data are mixed with other data. Labels are added here, as well. Setting up a proof of concept like this is a voyage of discovery, as it were. It involves examining source systems, target groups, the team of data scientists’ preference for certain tools, etc. All these things are then deployed within a proof of concept. Here data scientists will discover the various possibilities available. Take plenty of time for this. A proof of concept takes on average between three months and one year.
Step 3: Implement the data lake
In the next step, the data lake is actually implemented. The important thing here is to carry out the implementation step by step. All too often, the mistake is made of wanting to take account of every possible scenario in advance. But data lakes are so big and so complex that this will never work. The crux is to delineate and expand the scope slightly each time. This requires an agile way of working. Something new is learnt each time, data are enriched and thus become more complete. Security and compliance must be central during the implementation phase. It is important to think carefully about who can access what information and what data are to be added to the data lake. To put it simply: the more anonymous the data, the fewer measures have to be taken to be secure and compliant. Access management and encryption possibilities help here. As do conducting pen tests and grouping data.
Step 4: Create structured data
Some business needs can be met very well with the help of unstructured data from the data lake. But to carry out trend analyses, for example, structured data are necessary. In that case, data are taken from the data lake and, with a help of a tool such as Apache Hadoop, converted into information that can be used in a data warehouse. In fact, a subset of information is taken from the huge mountain of data in the data lake, which is then stored in an optimised and efficient database: the data warehouse.
Data lakes and data warehouses both meet a need, fitting in with one another. There will always be a demand in organisations for both structured and unstructured data, whereby the structured data consist of selected and processed unstructured data. By opting exclusively for a data lake, an organisation does not get the most out of the data, while with a data warehouse only, there is too little flexibility to be able to respond to the business’s changing needs. By combining the two, organisations get more out of their data.